Gemini amplifier. (Image: STFC, Clear Laser Facility)
Technological advances in high-power laser systems have given rise to the fast-growing and dynamic research field of laser-driven accelerators and radiation sources. Experimental and theoretical work on these topics advanced rapidly with the development of chirped-pulse amplification for lasers, which shared the 2018 Nobel Prize in Physics. This allowed for short duration laser pulses to be amplified and focused to such high intensities that electrons are stripped from their parent nuclei and oscillate at close to the speed of light in the laser fields.
At such high intensities, interactions are highly non-linear, meaning that small changes to the initial conditions can produce dramatically different results, often in ways which are difficult to predict or fully model. In experiments of this kind, there are many parameters in the laser system and interaction geometry that can be tuned to change the performance. Many of these can be also interdependent, adding to the complexity of interpretation. With so many dimensions to explore it is unlikely that the correct set of initial parameters will be chosen by chance, and so optimisation becomes very slow. In this situation it is advantageous to borrow from the on-going machine learning revolution and let computers do the hard work for us.
This is what an international team of researchers did in an experiment at the Central Laser Facility in the UK.
Firstly, they developed the technological capability to perform high intensity laser interactions at a relatively high repetition rate (5 shots per second). Then they gave a genetic algorithm control of the laser pulse shape and programmed it to optimise the x-ray source. After 30 minutes the algorithm had more than doubled the x-ray yield from the experiment, by optimising the heating process in a target of clustered argon gas. Following the experiment, it took significantly longer to figure out what the algorithm had done and why!
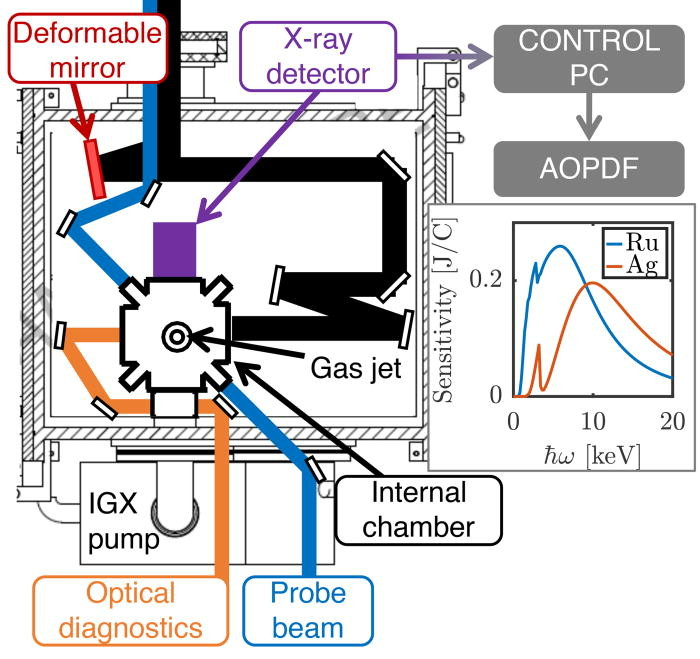
The results of this experiment, just published in Applied Physics Letters, show that the algorithm converged towards a laser pulse temporal shape with an initially gradually rising intensity ending with a sharp peak in intensity and rapid drop. In this experiment, the laser pulse was focused onto a jet of clustered argon, which are very efficient absorbers of laser radiation, once they have expanded. However, this expansion process requires some time and so a gradual rising edge allows for this. Then, once the conditions are optimal for laser absorption, the high-intensity spike arrives and rapidly heats the plasma and leads to increased x-ray production.
This work is the first attempt at applying machine learning techniques to such high intensity laser interactions, building on previous work with lower power but higher repetition rate laser systems. This approach allows for rapid exploration of a high-dimensional parameter space, without requiring prior understanding of what combination of parameters might be advantageous. As well as increasing the performance of the system, it also gives a new tool for understanding the physics of these systems by analysing the reasons behind the improved performance. Furthermore, active feedback techniques can also be used to stabilise performance of some system to a nominal level, adjusting laser parameters to compensate for changes in environmental conditions or aging of components. It is clear that use of such techniques will be of key importance in future experiments of this kind, and in much larger scale projects to build national and international scale facilities based on laser-plasma acceleration.
Further information: http://aip.scitation.org/doi/10.1063/1.5027297